AI Cures the Healthcare Data Gap
Editor's Note: Hospitals are continuously under pressure to increase medical staff capacity while keeping them safe and healthy. Today’s global healthcare environment is bringing this challenge to the forefront—particularly in the ICU. New technologies are helping them provide better care, while also caring for themselves.
Medical Informatics Corp. (MIC) is leading the way with solutions that enable clinical distancing while providing the best possible care. For example, its critical care platform hospitals can create virtual ICUs with centralized monitoring for up to 100 patients on a single screen—across vendors, units, and facilities.
Clinical staff can access data faster to manage ventilated patients better and avoid intubation. And they can view cardiac monitoring and vent data from different vendors in one integrated view. These are a few examples of how the MIC Sickbay Precision Medicine Platform for Critical Care is helping medical staff provide better care—under all kinds of circumstances. Read on to learn more.
What if patient diagnostic challenges could be solved with a single architecture, one that could unify data, improve monitoring, and eliminate data entry problems?
On a financial level, it would be easier for hospitals to get reimbursed. On a patient level, it could enable a new approach to precision healthcare: a practice that uses data to tailor treatments and decisions to improve outcomes.
Why does this matter? Heather Hitchcock, Chief Commercialization Officer at Medical Informatics Corp., explained: “The average ICU patient is connected to eight monitoring devices, generating up to 873,000 samples per hour. For example, cardiac monitors and ventilators can produce hundreds of different signals.”
Yet these devices were not designed to deliver all of this information to an electronic health record (EHR) or other care setting for clinical use. Instead, they transmit only a periodic snapshot. As a result, medical professionals receive on average less than 0.1 percent of total bedside patient data to make clinical decisions. Doctors and nurses are forced to rely on manual entry, taking time away from interacting with patients while increasing the risk of errors.
Data storage is another issue. These devices were designed to be monitored in real time. The ones that store data do so for a limited time—typically 24-72 hours. And often data is lost when the device is disconnected or turned off. This means the opportunity to collect, aggregate, and analyze patient information is also lost.
Solving Data Challenges with Real-Time Analytics
Medical professionals need a solution that allows them to discover patterns in patient response to treatments—enabling more informed decisions.
The Sickbay Precision Medicine Platform for Critical Care addresses that need. Developed by Medical Informatics Corp. (MIC), the solution automates real-time collection, aggregation, and time synchronization of data from bedside and other devices (Figure 1).
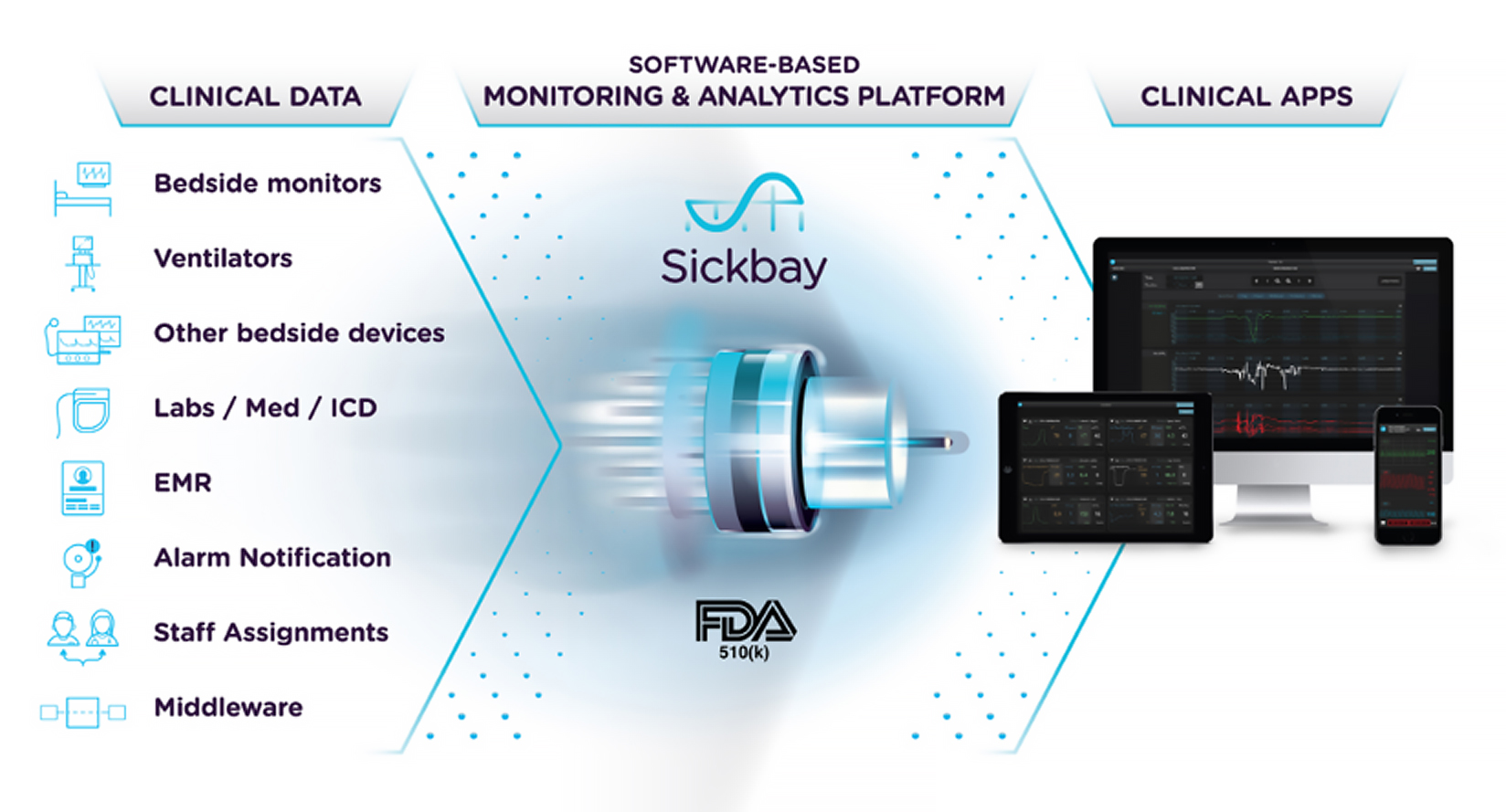
Lack of data and the ability to share it makes precision medicine nearly impossible. Everyday care becomes a problem for hospitals and clinics spread across a wide geography. For example, a hospital may have only one cardiologist who serves all sites. In such cases, the doctor might have to travel hours just to view a patient’s data. Yet the situation calls for a split-second decision.
The Sickbay platform makes it possible for the entire care team to access the same data, independent of their location, while also complying with regulations. It uses web-based applications and software that support vendor-agnostic remote monitoring, virtual ICUs, and high-fidelity waveform access to enhance documentation and manual processes.
The aggregated data can then be leveraged to improve response times and help identify risk factors in an individual patient. This can be done by using artificial intelligence (AI) and machine learning (ML) to analyze individual patient high-fidelity time series data along with EHR information, labs and medications, and more.
As a result, patterns related to illnesses, treatments, behaviors, and outcomes may be identified. For example, the solution can automate risk score calculations, provide patient trajectory monitoring with histograms, or deploy real-time predictive monitors at scale.1
“AI is not making decisions here. Instead it’s taking all those variables from all those devices and automating the calculation and processing of that data,” said Hitchcock. “It does it faster than humanly possible—producing results to help inform and expedite intervention and improve care.”
And while electronic medical records (EMRs) were intended to solve a multitude of data problems, they’re geared more to reimbursement than treatment. These records provide valuable information that supports AI, but they include only a fraction of the data needed.
“In the US, 90 percent of hospitals can only get waveform data into the EMR by printing it out on a strip, taping it to a sheet of paper, and scanning it,” said Hitchcock. “This wastes time and can introduce errors—compromising data.”
An Open Framework
Sickbay’s solution architecture enables a hospital to use one platform to handle all of its data needs at scale. That includes use cases such as automating the intake of waveform data as well as virtualization, risk scores, alarm management, and other processes.
And it does this on an open-source framework. Hospitals, vendors, and analysts can use real-time aggregated data to create applications and predictive analytics through open APIs and SDKs.
MIC creates additional value by developing apps in collaboration with different healthcare organizations. They can employ the solution to build apps for their own use or commercialize them on MIC’s app store (Figure 2).
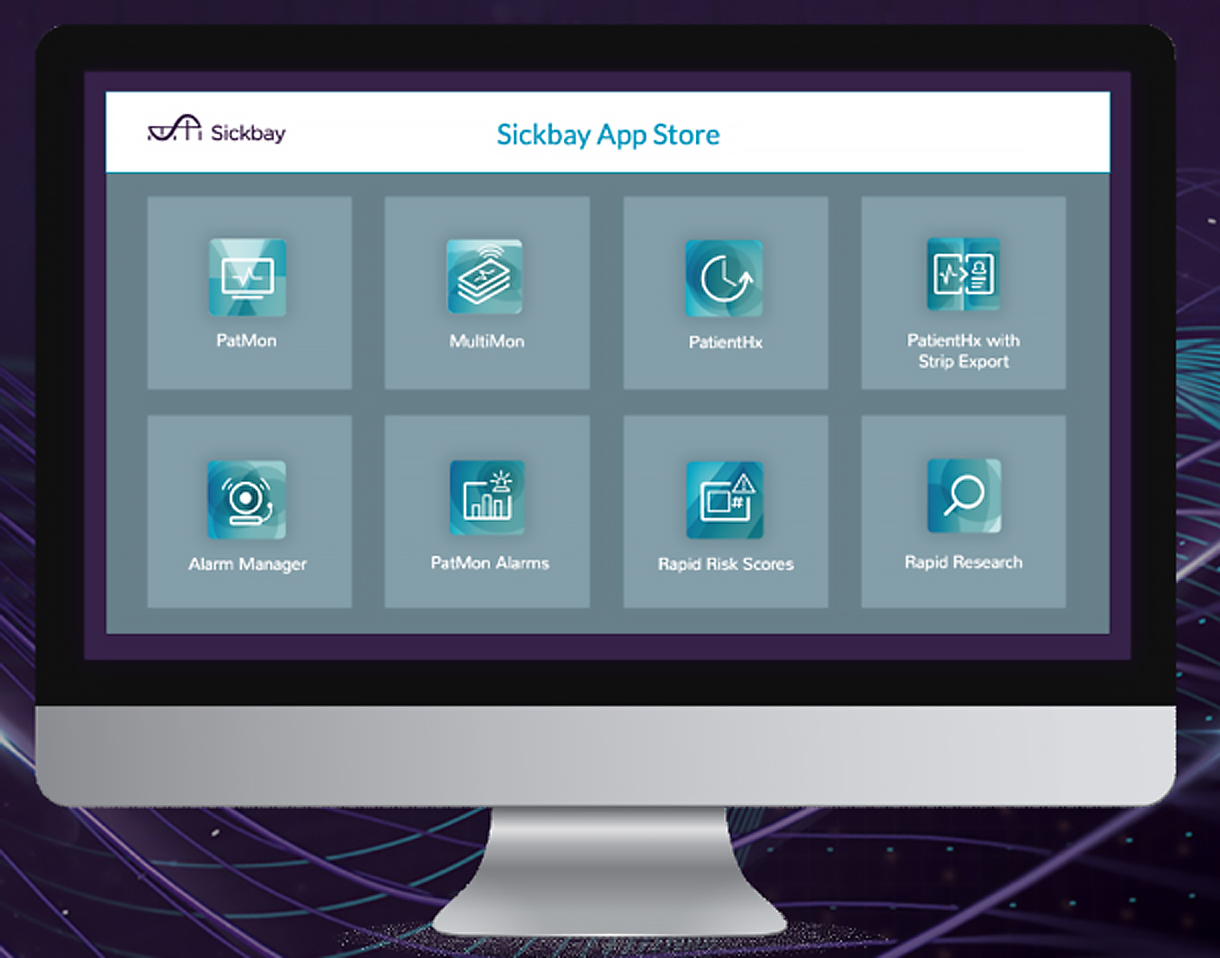
“With our open architecture, it’s not a one-trick pony that can solve only one problem,” said Hitchcock. “You plug it in, open your first app. Then add a new one, simply by turning on different database tables in the back end.”
AI at the Edge and in the Cloud
Hospitals need intensive compute power, performance, and security at the edge. “Intel® processors solve that need,” said Hitchcock. “But everything does not have to be on-site.”
While many hospitals will choose an on-premise solution, a hybrid cloud option can help lower costs, increase scalability, and gain greater insight from data, all while complying with HIPAA. Data acquisition servers can be located on-site and conduct machine learning locally. Results could be pushed to the cloud, where multiple hospitals can pool AI data.
For instance, a pediatric hospital that performs rare surgeries—such as treating single-ventricle babies—has a low sample size, making the amount of data too small to build an accurate AI training model.
But when multiple hospitals share data, there may be enough to analyze. Now they can use their results to carry out research or develop a software-based monitor. This opens the possibility to predict the onset of cardiac arrest in a single-ventricle baby before it occurs.
As new technologies deliver comprehensive real-time analytics, medical professionals and their patients can experience improved outcomes. The Sickbay Precision Medicine Platform for Critical Care offers promise in that direction.